I presented recent work on exploring the coherence of topics in fake news articles, at this year's International Workshop on News Recommendation and Analytics (INRA, held in conjunction with the ECML PKDD conference). This post summarises the work, its results and its potential impact. If you'd like to jump straight to the presentation, scroll down to the end of this page.
What is fake news?
Fake news (and more broadly, disinformation) is a pernicious and topical problem. But what is fake news? Well, it is one of the many kinds of disinformation we encounter nowadays. Any piece of information that is intentionally, and verifiably false, can be called fake newsDue to the frequent (and often suppressive) misuse of the term fake news, some researchers and journalists prefer to use mis- and disinformation.. Though, what makes a content fake, why it is shared, and the medium used to spread it varies.
People generally refer to false content as ‘fake news,’ but there are many different types of false content. Information produced or falsified, to deliberately manipulate, deceive or mislead people is called disinformation. On the other hand, information that is incorrect or not factual shared without malicious intent (but which could be perceived by the reader as true) is called misinformation. You can read more about mis- and disinformation in this blog post.
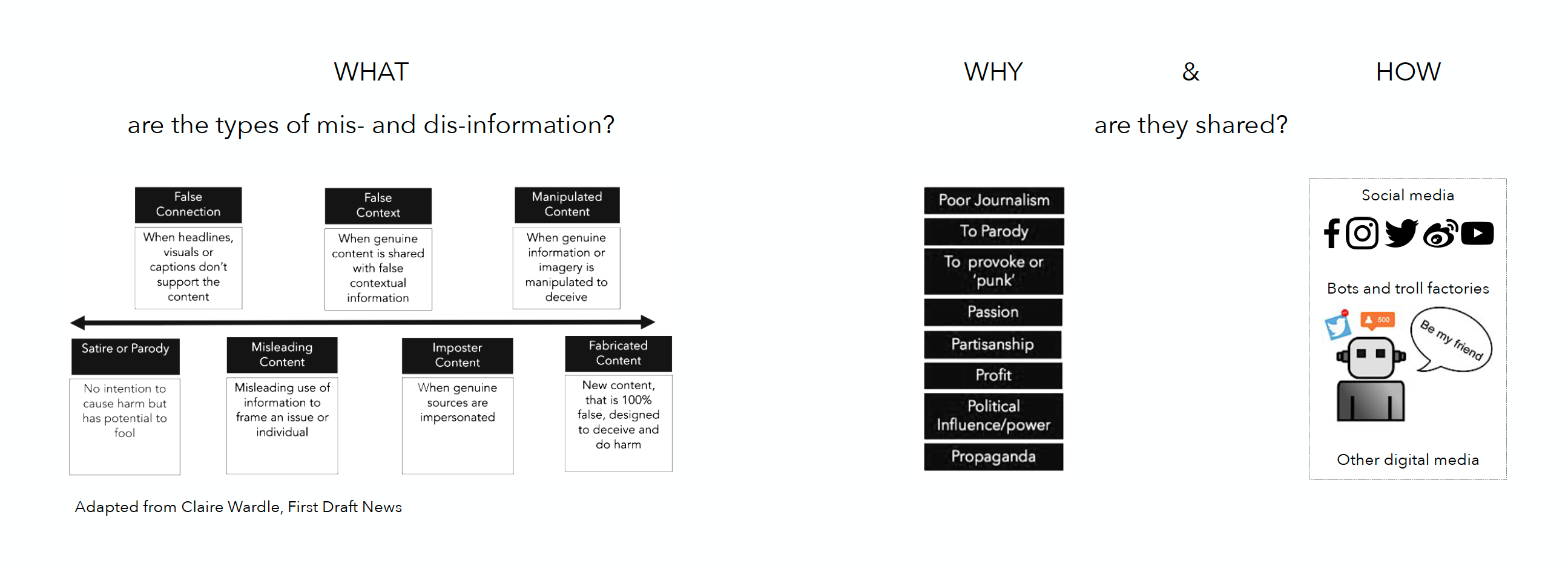
One of the motivations for our work comes from studies which show that people tend to share or even comment on news and other content online without reading the entirety of it. This allows bad actors to mislead by making the title or the opening section of an article enticing, and then enlacing latter sections of it with mis- or disinformation information. Such an article may be completely fabricated, shared using many bots on a social network, and used as a conduit for serving profit-making adverts.
Characterisation and detection
Our work focused on characterising fake news. Fake news, especially on social media, can spread and cause harm within a short time. Therefore, it is critical to intervene in its dissemination, as early as possible. Algorithms are more efficient for this task as compared to humans.
Research in creating algorithms for characterising and detecting is growing. The design of detection algorithms is informed by known characteristics of fake news, which come from diverse fields of research. For example, confirmation bias, which has its foundations in psychology, explains why people may hastily share news that agrees with their pre-existing beliefs or biases. Another example is malicious accounts on social networks, such as bots, which often exhibit a pattern of behaviour that differs from that of a real person. Fake news detection algorithms make use of the news content (for example, by comparing the writing styles of fake and real news), or social context (for example, by analysing how an article spreads through a social network). Creating a fake news dataset is difficult — it is time-consuming and can be expensive. Therefore, researchers need to make the most of available datasets.
Our research
Within existing datasets, text is the most abundant type of information. Our research aimed to come up with a new way of characterising fake news using text data. Existing methods of characterising fake news using text include analysing lexicon, syntax, or other features such as word or sentence embeddings. Characterisation informs and normally precedes the design of fake news detection algorithms. It is particularly key for creating unsupervised learning models. The more quintessential the characteristics are, the more robust an algorithm based on them will be.
Objectives
In this work, we set out to characterise fake news using topic modelling. We wanted to know whether the consistency of topics discussed in an article can be used as a high-level marker for spotting fake news. The intuition behind this is that fake news may contain a mix of topics that are not consistent throughout the article because the motivation is not to inform the reader, but rather, to mislead, draw their attention to ads on the page, or some other purpose for personal gain.
Method
Essentially, we used topics latent in an article to analyse how coherent its opening section is compared to the rest of it. We do this in three steps:
- The first step was to build the topic model. All articles in a given dataset, fake and real, are gathered into a corpus. The corpus was pre-processed to form a bag-of-words representation of each article. This data was then used to create an LDA model which learns topics from the data. ranged between 10 and 200.
- In the second step, we split each article into two parts: its opening paragraph or section; and the rest of it. The first five sentences were taken to represent the opening section because we think the introduction to an article is sufficiently captured in this section. We then pre-processed each section of the article like before.
- In the final step, we used the topic model to extract topics from both sections of the text and then calculated the deviation between the two topic distributions.
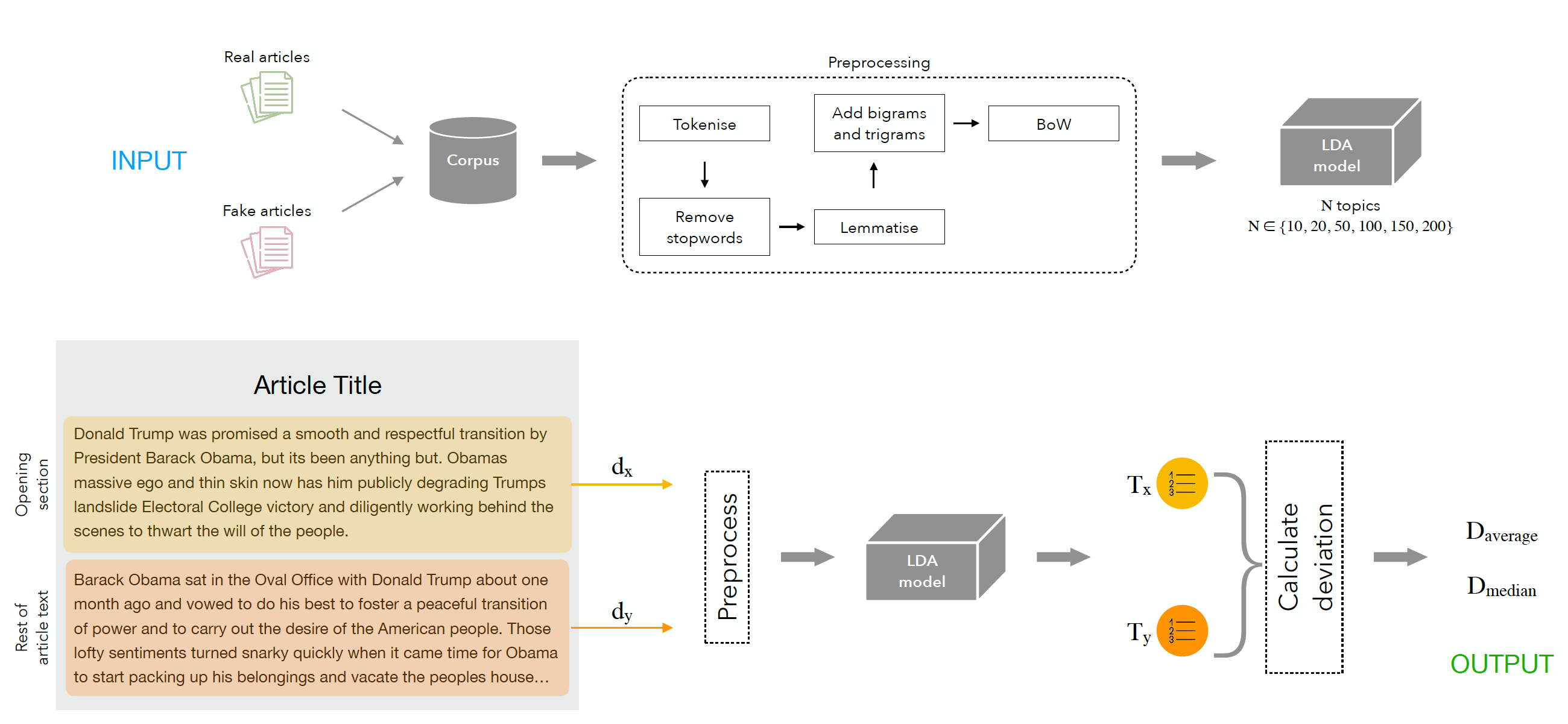
Experimental evaluation
We experimented with seven datasets of varying sizes. They also varied in the types of articles they contain, and collectively covered a broad range of themes—from politics to sports, to celebrities, etc.
The distance metrics we used were the Chebyshev (Chessboard), Euclidean and Squared Euclidean distances. To determine if there is a significant difference between the coherence or fake and real news, we used the T-test statistical test
Results and observations
We found that the mean and median deviation of fake news is greater than that of real news in all datasets and for all metrics. Our results also showed statistical significance at the level for all but two datasets. This suggests that it may be possible to characterise fake news based on how the topics it discusses shift through the article.
Paper
The full paper is here (and also on arXiv). Our next step is to build on these characteristics, to develop unsupervised models for fake news detection.
How to cite:
Dogo M.S., Deepak P., Jurek-Loughrey A. (2020) Exploring Thematic Coherence in Fake News. In: Koprinska I. et al. (eds) ECML PKDD 2020 Workshops. ECML PKDD 2020. Communications in Computer and Information Science, vol 1323. Springer, Cham. https://doi.org/10.1007/978-3-030-65965-3_40